Virtual screening involves the use of in silico methods to screen for potential small molecule binders of a target protein. This process usually starts with computationally docking a virtual library of chemical compounds to assess how the compounds interact with a defined binding site on the target protein. This is followed by scoring to predict the approximate binding affinity of these compounds to the target.
Through virtual screening, computational chemistry has an unprecedented opportunity today to re-shape traditional drug discovery’s heavy dependence on lab-based screening approaches. One of the exciting developments for the field was the recent availability of over 200 million 3D protein structures predicted by Deep Mind’s AlphaFold. In parallel, virtual chemical libraries have been rapidly expanding, like Enamine’s commercially available make-on-demand “REAL (REadily AccessibLe) Space”, which provides the potential of accessing up to 31 billion compounds. With recent developments in computational approaches such as machine learning, it is now possible to integrate AI with traditional physics-based modeling to allow the identification of novel and chemically tractable hit compounds for any protein target.
The computational chemistry team at EDDC has been running ultra-large-scale virtual screening as a hit-finding approach for a series of targets. We learnt that a reasonable hit rate can be achieved when virtual screening is applied with careful druggability assessment, followed by a good compound selection process. Virtual screening works best with targets like kinases and GPCRs that have well-defined structural data. However, when there is insufficient structural data for a target protein, a common alternative is to work with homology models instead. Below, we share two case studies of projects where EDDC’s computational chemistry team applied these techniques.
Case study 1: WLS (Wntless)
Wnt signalling molecules are lipid-modified glycoproteins that play key roles in both embryonic development and adult homeostasis. Its signalling cascade is dysregulated in many cancers and emerging pre-clinical data supports that targeting Wnt biosynthesis is effective in Wnt-addicted cancers. A protein known as WLS/Evi is essential for Wnt secretion. A newly solved Cryo-EM structure of WLS in complex with WNT8A shows that WLS has a druggable G-protein coupled receptor (GPCR) domain. In collaboration with Prof David Virshup, Director of the Programme in Cancer and Stem Cell Biology (CSCB) and Professor at Duke-NUS Medical School, and a leading expert in Wnt biology, we performed an ultra-large-scale virtual screen with 500 million compounds. 68 putative hits were obtained and tested in cell-based assays by Prof Virshup’s laboratory. After more refinement and biological testing, two compounds emerged as potential first-in-class WLS inhibitors.
Prof David Virshup shared, “Our collaboration with EDDC’s computational chemistry group was very productive. They first learned a lot about our target, providing valuable insights. We then generated testable hypotheses based on in silico homology modelling and virtual screening, and validated them at the bench. It was a great experience.”
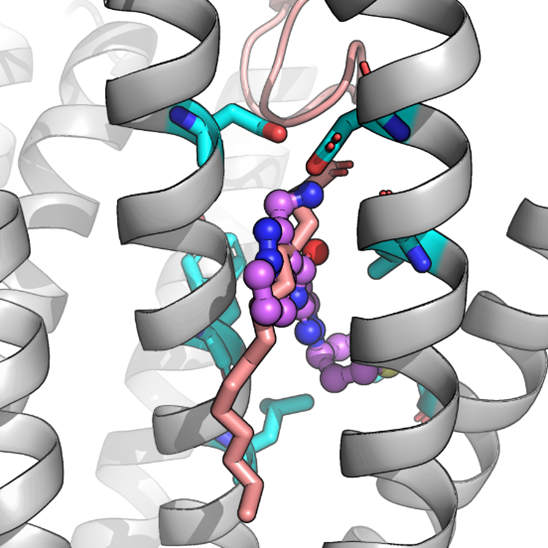
Figure 1. Predicted binding and inhibitory mechanism of a WLS inhibitor (purple) perturbing the binding of Wnt-8A (salmon).
Case study 2: Kinase Target X
There was a lack of structural information on this kinase target at the time of virtual screening – no crystal structure or AlphaFold structure was available. We constructed two homology models of the target -a crude model, which was built based on the template protein directly and a trained model docked by known inhibitors of the kinase target. This is then followed by molecular dynamic simulations a hypothesized active conformation of the protein-inhibitor complex. Both models were subjected to virtual screens. The trained model led to the discovery of two hits (IC50 < 10 μM) out of 36 compounds tested (5.6% hit rate), while the crude model did not result in any hits. This suggests that virtual screening may be applied to homology models if careful training of the model is done beforehand.
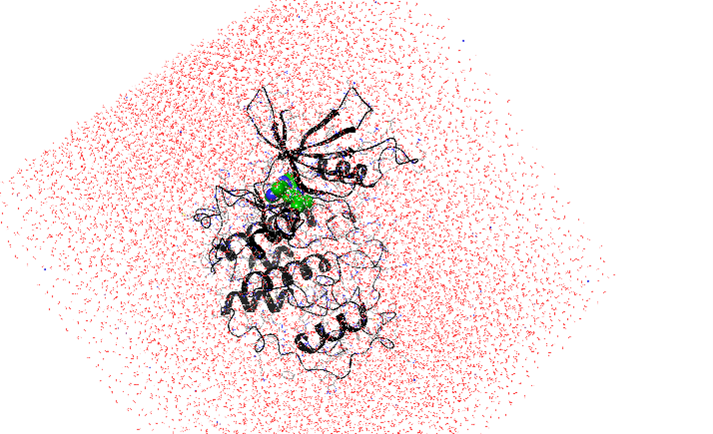
Figure 2. A simulation system containing solvated kinase homology model in complex with an inhibitor.
In addition to these two case studies, we have also worked on more challenging targets – for example, proteins that are highly flexible and have multiple conformations which affect the binding sites. We have looked at conducting virtual screening based on multiple static conformations, as well as running high-throughput or fragment-based screening in parallel to increase the chances of finding tractable hits.
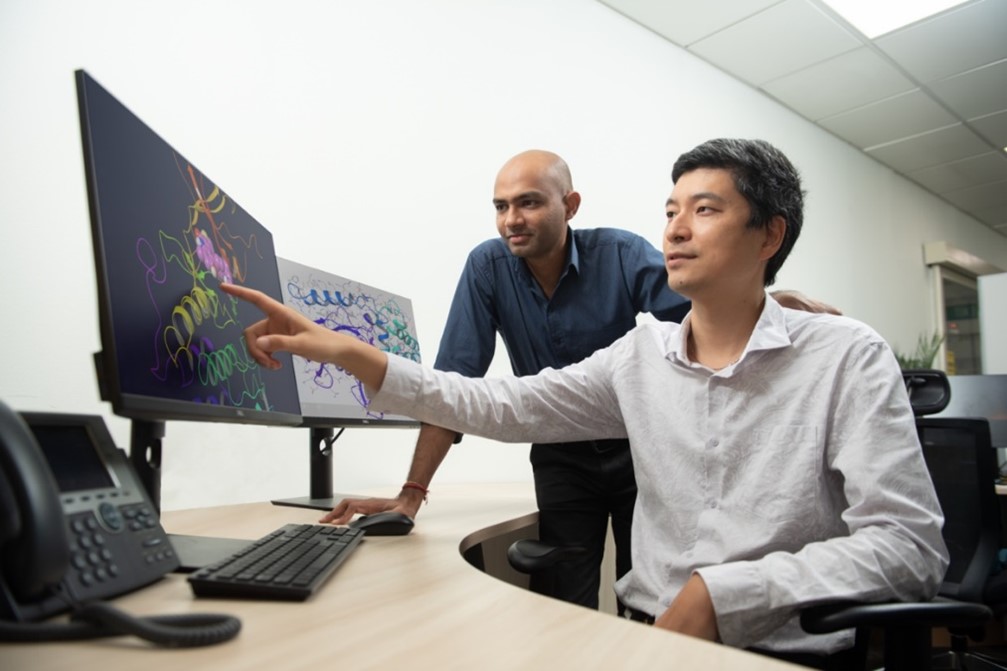
From left to right: Padmanabhan Anbazhagan and Xu Weijun. Computational chemists analysing data to plan for a screen.
Despite all the advantages of computational approaches, we believe that the application of these in silico techniques should be carefully monitored and statistically compared to experimental approaches on a project-by-project basis. It is also crucial to work with collaborators who have a deep biological understanding of the target. Ultimately, the accumulated experience from both successes and failures will help us to improve this discovery process for future targets.